Algorithmic and Non-Algorithmic Fairness: Should We Revise our View of the Latter Given Our View of the Former?
New publication by Kasper Lippert-Rasmussen in Law and Philosophy
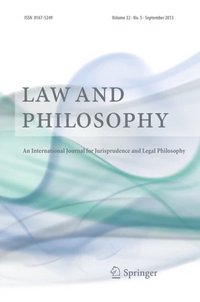
DOI: https://doi.org/10.1007/s10982-024-09505-4
Abstract: In the US context, critics of court use of algorithmic risk prediction algorithms have argued that COMPAS involves unfair machine bias because it generates higher false positive rates of predicted recidivism for black offenders than for white offenders. In response, some have argued that algorithmic fairness concerns, either also or only, calibration across groups–roughly, that a score assigned to different individuals by the algorithm involves the same probability of the individual having the target property across different groups of individuals–and that, for mathematical reasons, it is virtually impossible to equalize false positive rates without impairing the calibration. I argue that in standard non-algorithmic contexts, such as hirings, we do not think that lack of calibration entails unfair bias, and that it is difficult to see why algorithmic contexts, as it were, should differ fairness-wise from non-algorithmic ones in this respect. Hence, we should reject the view that calibration is necessary for fairness in an algorithmic context.